Case StudyStream Team: Running Experiments - Optimizing User Experience with A/B Testing at Scale
Booking.com, one of the world's leading online travel agencies, faced the challenge of continuously improving its user experience and conversion rates. With millions of users interacting with the platform daily, it was essential to make data-driven decisions to ensure that changes led to positive outcomes. Traditional product development methods were not sufficient to validate the impact of changes quickly and accurately. Booking.com needed a robust approach to test hypotheses and measure their effects on user behavior.
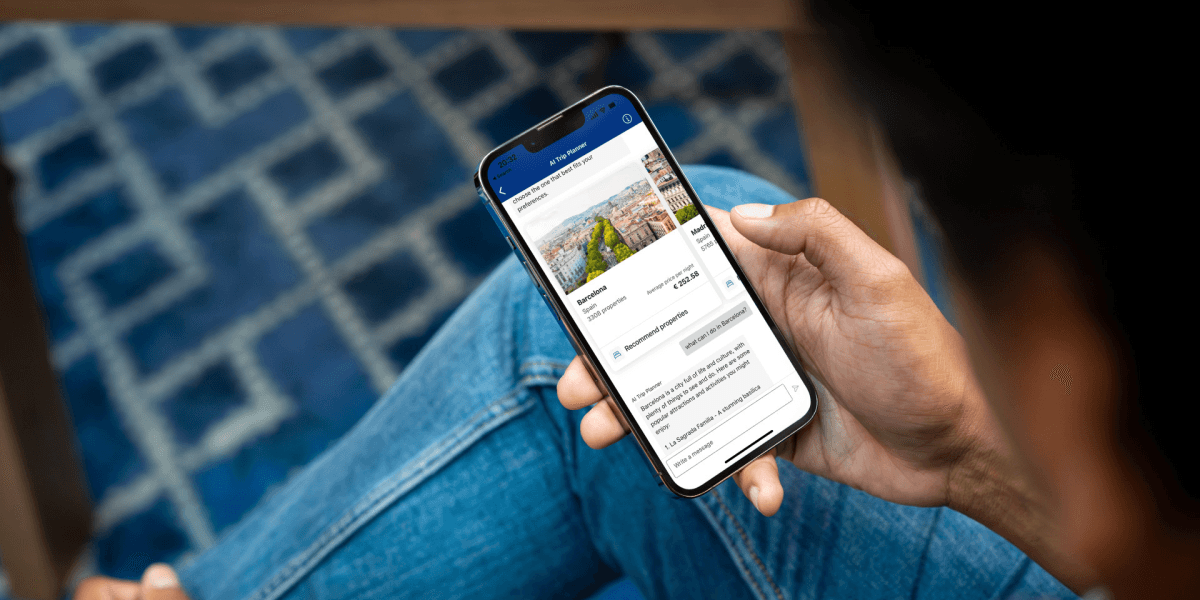
The Solution
Booking.com implemented A/B testing at scale as a core part of their product development strategy. The key components of their approach included:
- Hypothesis-Driven Testing: Each A/B test started with a clear hypothesis about how a specific change might improve user experience or increase conversions. This hypothesis-driven approach ensured that tests were focused and meaningful.
- Randomized User Segmentation: Users were randomly segmented into control and variant groups to ensure that the results were statistically valid and unbiased. This randomization helped in isolating the impact of the changes being tested.
- Robust Analytics Infrastructure: Booking.com invested in a robust analytics infrastructure capable of handling large-scale data collection and analysis. This infrastructure allowed the team to track user interactions and measure the impact of changes in real-time.
- Iterative Testing Cycles: A/B tests were conducted in iterative cycles, with each cycle building on the insights gained from previous tests. This iterative approach allowed for continuous optimization and refinement of user experience.
- Cross-Functional Collaboration: The A/B testing process involved cross-functional teams, including designers, developers, product managers, and data analysts. This collaboration ensured that all perspectives were considered, and that insights from tests were integrated into product development.
- Scalable Testing Framework: Booking.com developed a scalable testing framework that allowed multiple A/B tests to be run simultaneously across different parts of the platform. This scalability was crucial for testing numerous hypotheses quickly and efficiently.
Outcomes achieved
The implementation of A/B testing at scale led to several significant outcomes for Booking.com:
- Improved User Experience: Continuous A/B testing allowed Booking.com to make data-driven decisions that enhanced the user experience. By testing and validating changes, the platform was able to implement features that users found valuable and intuitive.
- Increased Conversion Rates: A/B testing helped identify the most effective changes for increasing conversions. By focusing on data-backed improvements, Booking.com achieved higher booking rates and customer satisfaction.
- Faster Innovation: The iterative nature of A/B testing enabled Booking.com to innovate rapidly. The ability to test and validate ideas quickly meant that successful innovations could be rolled out faster.
- Data-Driven Culture: The widespread adoption of A/B testing fostered a data-driven culture within Booking.com. Teams relied on empirical evidence to guide their decisions, reducing the risks associated with product changes.
- Scalable Optimization: The scalable testing framework allowed Booking.com to optimize various aspects of the platform simultaneously. This holistic approach ensured that improvements were made across the entire user journey.